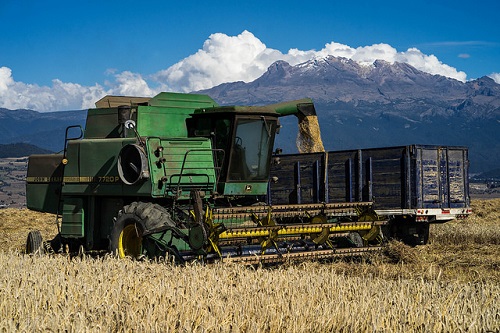
With increasing global demand for wheat and increasing constraints (high temperatures, diseases) to wheat’s productivity, wheat breeders are looking for new methodologies to make breeding more efficient. A new study looks at refinements of genomic prediction models to help achieve this.
The authors write that genomic selection is becoming a standard approach to achieving genetic progress in plants, as it gets around the need to field-test the offspring at every cycle, but that the models commonly used in plant breeding are based on datasets of only a few hundred genotyped individual plants.
This study used pedigree and genomic data from nearly 59,000 wheat lines evaluated in different environments, as well as genomic and pedigree information in a model that incorporated genotype X environment interactions to predict the performance of wheat lines in Mexican and South Asian environments.
They found that models using markers (and pedigree) had higher prediction accuracies than models using only phenotypic data. Models that included genomic x environment had higher prediction accuracies than models that do not include interaction.
Read the full study “Single-Step Genomic and Pedigree Genotype × Environment Interaction Models for Predicting Wheat Lines in International Environments” and check out other publications by CIMMYT staff below:
- Association mapping reveals loci associated with multiple traits that affect grain yield and adaptation in soft winter wheat. 2017. Lozada, D. N., Mason, E.R., Md Ali Babar, Carver, B. F., Guedira, G. B., Merrill, K., Arguello, M. N., Acuna, A., Vieira, L., Holder, A., Addison, C., Moon, D. E., Miller, R. G., Dreisigacker, S. In: Euphytica v. 213 : 222.
- Effect of trait heritability, training population size and marker density on genomic prediction accuracy estimation in 22 bi-parental tropical maize populations. 2017. Ao Zhang, Hongwu Wang, Beyene, Y., Fentaye Kassa Semagn, Yubo Liu, Shiliang Cao, Zhenhai Cui, Yanye Ruan, Burgueño, J., San Vicente, F.M., Olsen, M., Prasanna, B.M., Crossa, J., Haiqiu Yu, Zhang, X. In: Frontiers in Plant Science v. 8 : 1916.
- Genomic prediction unifies animal and plant breeding programs to form platforms for biological discovery. 2017. Hickey, J.M., Tinashe Chiurugwi, Mackay, I., Powell, W., Eggen, A., Kilian, A., Jones, C., Canales, C., Grattapaglia, D., Bassi, F., Atlin, G.N., Gorjanc, G., Dawson, I., Rabbi, I., Ribaut, J.M., Rutkoski, J., Benzie, J., Lightner, J., Mwacharo, J., Parmentier, J., Robbins, K., Skot, L., Wolfe, M., Rouard, M., Clark, M., Amer, P., Gardiner, P., Hendre, P., Mrode, R., Sivasankar, S., Rasmussen, S., Groh, S., Jackson, V., Thomas, W., Beyene, Y. In: Nature Genetics v. 49, no. 9, p. 1297–1303.
- Genomic selection in plant breeding : methods, models and perspectives. 2017. Crossa, J., PĂ©rez-RodrĂguez, P., Cuevas, J., Montesinos-Lopez, O.A., JarquĂn, D., De los Campos, G., Burgueño, J., Camacho-González, J. M., Perez-Elizalde, S., Beyene, Y., Dreisigacker, S., Singh, R.P., Zhang, X., Gowda, M., Rutkoski, J., Varshney, R. K. In: Trends in Plant Science v. 22, no. 11, p. 961-975.
- Single-step genomic and pedigree genotype x environment interaction models for predicting wheat lines in international environments. 2017. PĂ©rez-RodrĂguez, P., Crossa, J., Rutkoski, J., Singh, R.P., Legarra, A., Autrique, E., De los Campos, G., Burgueño, J., Dreisigacker, S. In: The Plant Genome v. 10, no. 2.