Artificial Intelligence (AI) and Machine Learning (ML) are increasingly being applied across a diverse range of disciplines. Many aspects of our lives and work are now benefiting from these technologies. Disease recognition, for both human and plant health, is no exception. Ever more powerful AI/ML techniques are now opening up exciting opportunities to improve surveillance, monitoring and early warning for disease threats.
Scientists from Penn State University/PlantVillage, working with CGIAR centers, FAO and national country partners, are at the forefront of AI/ML technology development applied to crop pest and disease recognition. Development of the “PlantVillage Nuru” mobile app has provided an accurate and simple automated disease diagnostic tool that can be used by non-experts, including farmers. A recent paper published in Frontiers of Plant Science demonstrated that Nuru could diagnose symptoms of cassava diseases at higher accuracy than agricultural extension agents or farmers.
“The value of tools like PlantVillage Nuru is that we can greatly increase the coverage and speed of surveillance,” says CIMMYT scientist and disease surveillance expert Dave Hodson. “Trained pathologists can only visit a limited number of fields at fixed times in the season. With tools like Nuru, extension agents and farmers can all contribute to field surveys. This can result in much faster detection of disease outbreaks, better early warning and improved chances of control”.
New advances in AI/ML technology are now promising even greater improvements in these already powerful tools. CIMMYT scientists have had a long-standing partnership with the PlantVillage group, working to try and develop improved diagnostics for important wheat diseases such as rusts and blast. Considerable progress in developing automated diagnostics for wheat diseases has already been made, but the introduction of advanced image segmentation and tiling techniques promises to be a major leap forward.
“Advances in computer science are constantly happening and this can benefit the mission of CGIAR and PlantVillage,” explains David Hughes, Dorothy Foehr Huck and J. Lloyd Huck Chair in Global Food Security at Penn State and founder of PlantVillage.
“Image segmentation and tiling techniques are a great example. They used to require intensive computing requirements. Now due to advances in computer science these powerful techniques are becoming more accessible and can be applied to plant disease problems like wheat rusts.”
By using these image segmentation and tiling techniques the developers at PlantVillage are now seeing a major improvement in the ability to automatically and accurately detect wheat rusts from in situ photos. “We could not identify rusts with the older approaches but this segmentation and tiling tool is a game changer. The computer goes pixel by pixel across the images which is well suited to diseases like rusts that can be spread across the leaf or stem of the plant. The computer now has a much more powerful search algorithm.”
The team led by Pete McCloskey, lead A.I. engineer at Plant Village, actually used a multi-step process. First they removed the background to help the machine focus in on the leaf. They then digitally chopped the leaf into segments giving the AI a further helping hand so it can focus in and find the rust. Then the whole leaf is stitched together and the rust is highlighted to help humans working in the PlantVillage cloud system.
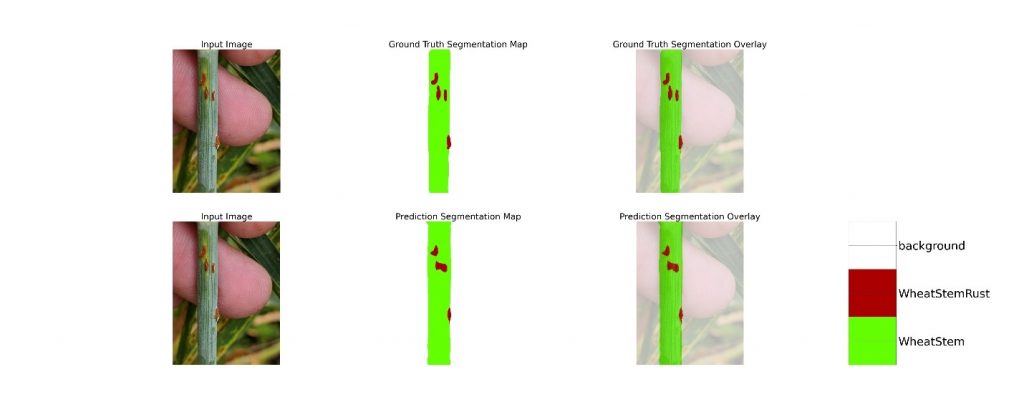
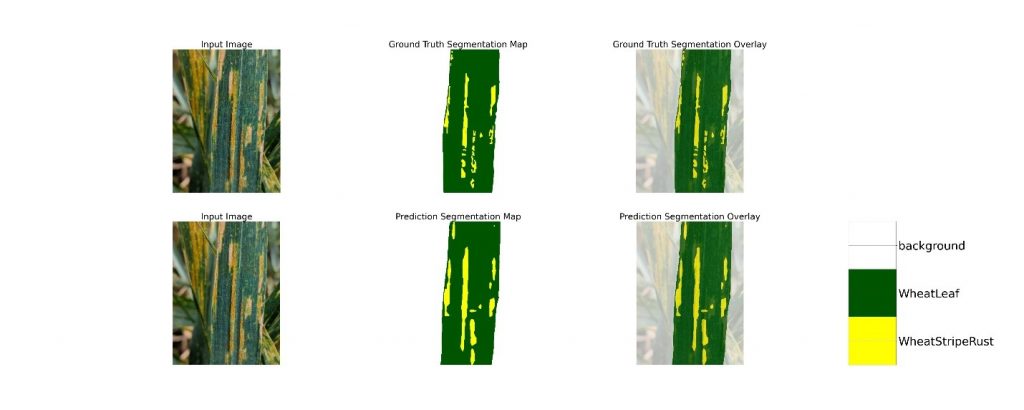
This exciting new development in rapid, accurate field detection of wheat rusts now needs validation and improvement. As with all AI/ML applications, numbers of images included in the models really improve the quality of the final predictions. “The success of any machine learning model is rooted in the quality and quantity of the data it is trained on,” notes McCloskey. “Therefore, it is critical to source vast and diverse amounts of high-quality images from around the world in order to develop a global wheat rust recognition system.” In this aspect we hope that the CIMMYT global wheat community can help drive the development of these exciting new tools forward.
CIMMYT and PlantVillage are hoping to expand the current wheat rust image dataset and as a result produce an even more valuable, public good, disease detection tool. Given the extensive field work undertaken in wheat fields around the world by CIMMYT staff and partners, we hope that you can help us. Any photos of wheat rusts (stem, stripe and leaf rust) in the field would be valuable.
We would like to have images with one infected leaf or stem per image, it should be vertical in the image so you can see the whole leaf or stem segment. The leaf or stem needs to be in focus and should be roughly centered in the image. It helps to hold the tip of the leaf away from the stem, so it is outstretched and flat. Ideally for training data, the leaf should have only one type of rust and no other disease symptoms. It is okay to have other leaves/stems/soil/sky in the background. It is also okay to have hands and other body parts in the image.
Below are some example images. Any images can be uploaded here.
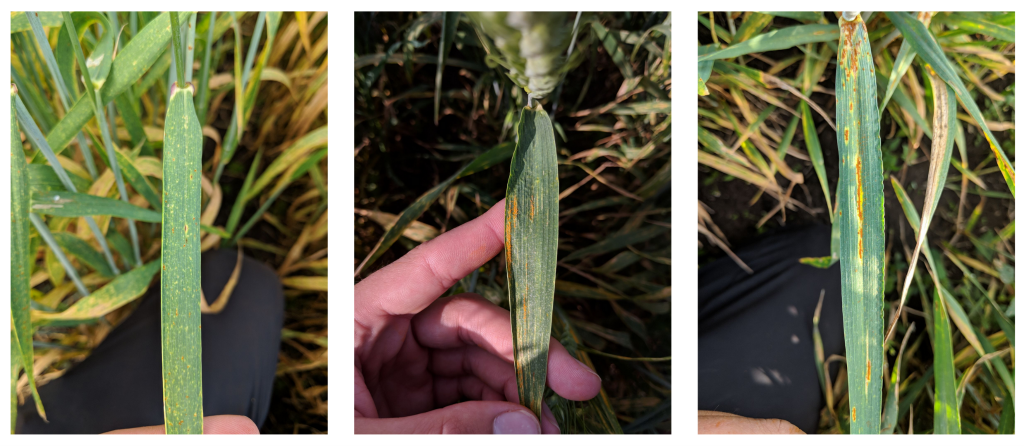
For more information contact Dave Hodson, CIMMYT (d.hodson@cgiar.org) or Pete McCloskey, PlantVillage (petermccloskey1@gmail.com).Â